

MethylationToActivity (M2A)
Read about my most recent project, or keep scrolling to learn more about me.
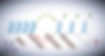
READ THE PAPER
*Currently under review and available at BioRxiv
MethylationToActivity is a new method to infer histone modification enrichment (including H3K27ac and H3K4me3) from DNA methylation data.*
Trained from pediatric neuroblastoma samples, we show our model is highly generalizable across cancer types and approaches accuracies of actual ChIP-seq experiments.
MethylationToActivity:
a deep-learning framework that reveals promoter activity landscapes from DNA methylomes in individual tumors
RUN M2A ON THE CLOUD

Justin Williams
Postdoctoral Data Scientist
St. Jude Children's Research Hospital
(724) 833-3414
About
MY BACKGROUND
My name is Justin Williams. I am a Postdoctoral Data Scientist in Memphis, TN using machine learning to better understand childhood cancer. Although my training during graduate school was plant-based, I have long held an interest in gene regulation and epigenetics in cancer systems. Not surprisingly, many of the concepts and skills I learned during my PhD studies were transferrable, which allowed me to join the Chen Lab in the Computational Biology Department at St. Jude Children's Research Hospital.
I learned that computational biology is what I am most passionate about and where I can make the biggest impact. My main goal is to generate a model that can accurately, generalizably, and efficiently predict drivers of cancer gene (de)regulation in patient samples. Broadly, there are two confounding aspects of this goal. The first aspect is also part of the solution; with ever increasing sample sizes, the increase in data dimensionality raises concerns in terms of computational storage, run-time, and cost. Secondly, is the lack of functional understanding of complex biological signals, and their potential impact on phenotype.
​
My approach to solving the intersection of these problems lies in machine learning; a concise way of representing a large amount of data, which allows researchers and clinicians to fully understand the functional impact of biological marks, such as DNA methylation.
Skills & Languages
WHAT I BRING TO THE TABLE
FAMILIAR
PROFICIENT
Data Analysis
Machine Learning
Cancer Biology
Wet Lab Techniques
Sequencing Technology
Biochemistry
Image Analysis
Python
R
